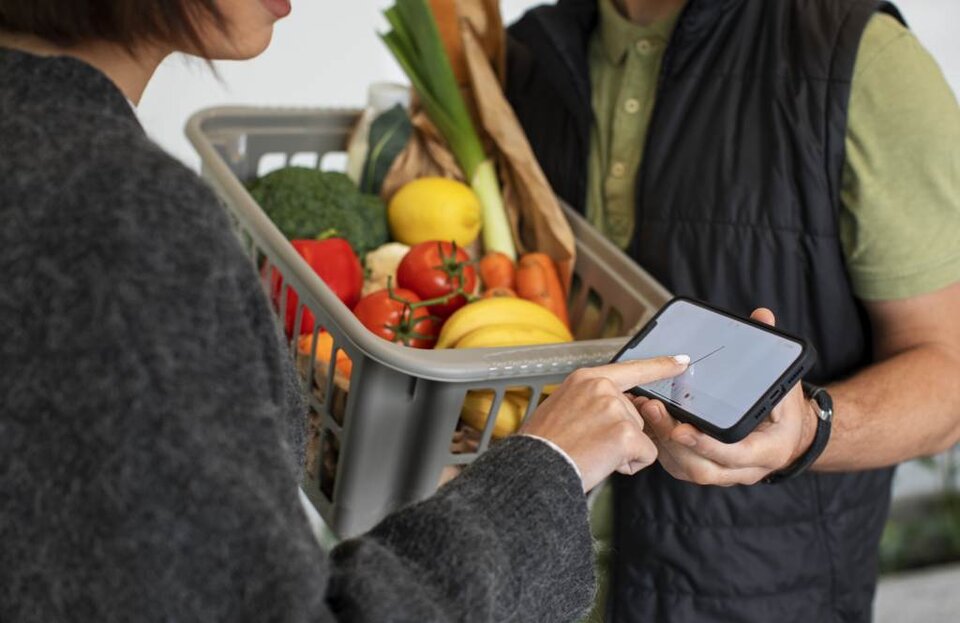
Using AI and Predictive Analytics to Solve Retail’s Returns Challenge
As eCommerce continues to grow, retailers face increasing pressure to offer flexible, efficient returns processes while maintaining financial viability. Many retailers are adopting strategies to streamline returns and leveraging AI tools to reduce return rates and improve customer satisfaction.
One key approach is simplifying the returns process to make it hassle-free, offering easy return labels, clear policies, and multiple options such as in-store, mail, or drop-off points. Additionally, real-time inventory management allows retailers to quickly reintegrate returned products into stock, minimizing downtime and reducing the risk of obsolescence. Optimizing reverse logistics—such as inspecting, refurbishing, or recycling returned goods—further maximizes value and reduces waste.
Understanding why customers return products is also crucial. Analyzing return data helps retailers improve product descriptions, quality control, and fulfillment accuracy, which can lower return rates.
AI plays a significant role in addressing these challenges. Predictive analytics identify products with higher return rates, allowing retailers to improve product quality and inventory management. AI-driven virtual try-ons and fit technologies help customers make better purchasing decisions, reducing returns due to sizing issues. Automated returns processing speeds up the workflow, and AI-powered damage detection ensures that fewer incorrect or damaged items are shipped.
By combining these strategies with AI tools, retailers can improve the efficiency of their returns process, reduce overall return rates, and boost customer satisfaction—leading to increased loyalty and repeat business.
