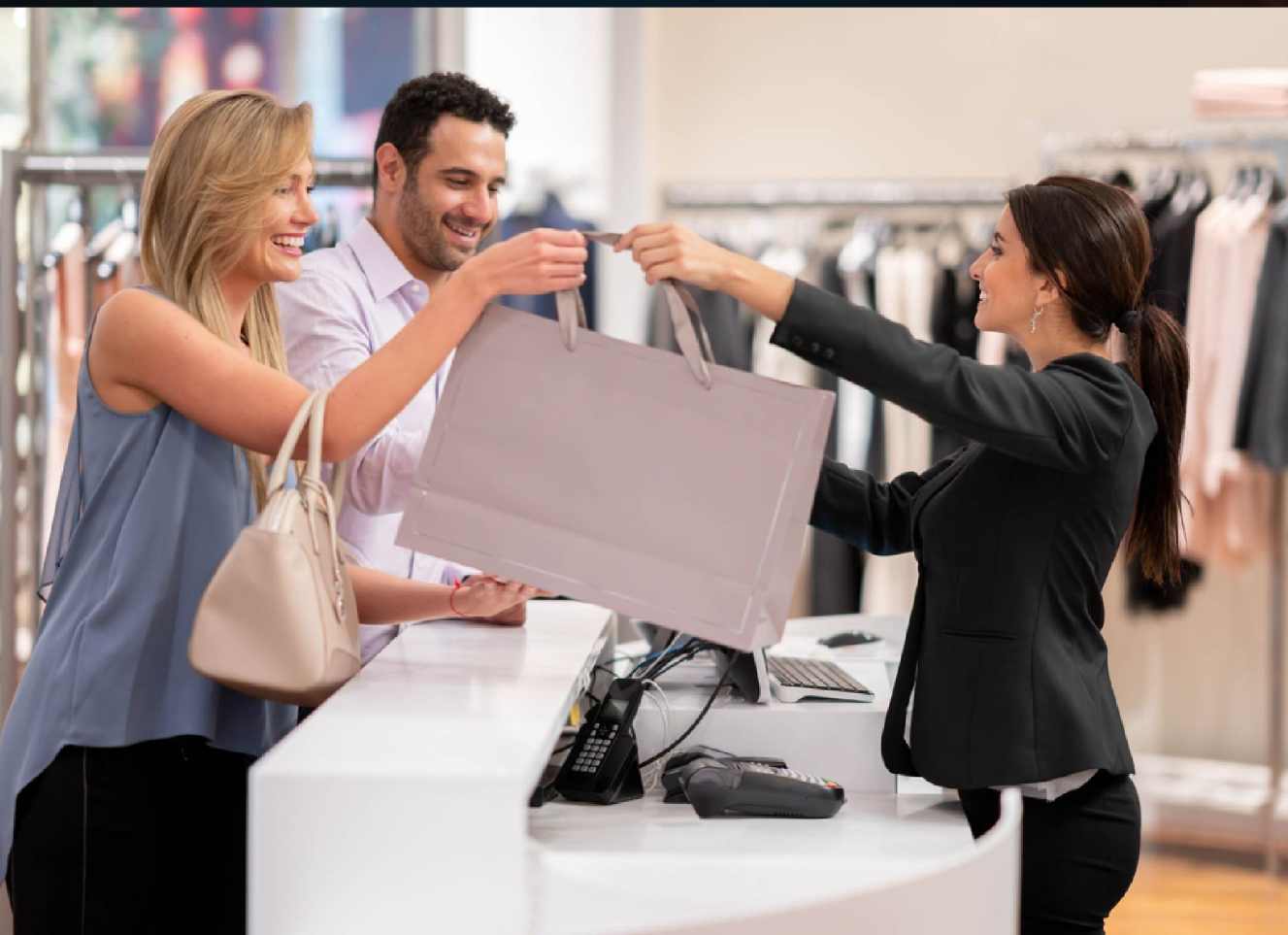
AI & Store-Level Insights for Strategic Decision- Making
Revolutionizing Retail:
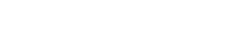
HIVERY
HIVERY is a pioneer of next-generation retail assortment strategy simulation & optimization analytics. These proprietary machine learning and applied mathematics algorithms were co-developed and acquired from Australia’s national science agency - CSIRO’s Data61.
HIVERY was founded on the vision that Data Has A Better IdeaTM - and we’re working together with our clients to uncover its full potential.
INTRODUCTION: The Evolution of Retail and the Rise of AI
The retail industry has undergone significant transformations over the past few decades. From the traditional brick-and-mortar stores to the advent of e-commerce and now the integration of artificial intelligence (AI) and advanced analytics, the retail landscape continues to evolve at an unprecedented pace.
In the early days, retail was a straightforward process. Consumers visited physical stores, selected products from the shelves, and completed their purchases at the checkout counters. Retailers used simple performance metrics such as total sales or rotation numbers to manage their stores. However, this approach had its limitations. Understanding customer behavior, preferences, and shopping patterns was challenging, which is crucial for optimizing store performance and enhancing customer satisfaction.
The advent of e-commerce brought about a significant shift in the retail industry. Online shopping platforms offer consumers the convenience of browsing and purchasing products from the comfort of their homes. Retailers had access to a wealth of data, from browsing history to purchase patterns, enabling them to personalize the shopping experience and improve their service offerings.
However, this data's sheer volume and complexity presented new challenges. Traditional data processing and analysis methods proved inadequate in extracting meaningful insights from this vast pool of information. This is where AI and advanced analytics came into the picture.
AI has revolutionized the way retailers process and analyze data. With the ability to learn from data, AI can identify patterns and trends, make predictions, and provide actionable insights. Advanced analytics, on the other hand, allows retailers to delve deeper into the data, uncovering granular insights that were previously inaccessible.
Today, AI and advanced analytics are optional extras and essential tools for any retailer looking to stay competitive. They offer the ability to leverage store-level data, providing a comprehensive analysis of customer behavior at the push of a button. This capability transforms various aspects of retail, from SKU rationalization and product innovation to assortment and category strategies.
As we delve deeper into this report, we will explore how AI and advanced analytics empower retailers and CPG suppliers to collaborate more effectively, make strategic decisions, and ensure they have the right product in the right place at the right time. The future of retail is here, and AI powers it.
The Power of Store-Level Insights: Understanding the Potential
In the retail industry, the mantra "location, location, location" has evolved into "data, data, data." AI and advanced analytics have ushered in a new era where store-level insights are valuable and indispensable for success. These insights, derived from granular data at the store level, provide a comprehensive understanding of customer behavior, preferences, and shopping patterns, enabling retailers and CPG suppliers to make informed, strategic decisions.
Store-level data goes beyond performance metrics such as total sales or rotation numbers. It delves into the intricate details of customer behavior, capturing the nuances of shopper preferences at individual stores. This granular level of data allows for a more precise and targeted approach to assortment planning, SKU rationalization, product innovation, and other category strategies.
The retail landscape is undergoing a seismic shift, with AI and advanced analytics at the helm. McKinsey's report1 underscores the transformative potential of these technologies, revealing that tasks once considered unsuitable for automation, such as “applying expertise” and “collaboration” between humans and systems, are now achievable. These two “tasks” collectively, the shift could lead to a staggering over 100 % productivity gain, effectively doubling current industry capabilities and speed to market value.
One of the critical areas where this transformation is evident is in the realm of store-level insights. A case study involving ten stores illustrates the power of AI in validating intuitive hunches and driving strategic decisions. The experiment revolved around the behavior of a specific shopper segment, the "domestic premium shopper" at a popular convenience retail store chain. The hypothesis was that these shoppers were primarily focused on seeking the lowest prices and were less concerned with whether the product was on a warm or cold shelf. Moreover, it was believed that premium shoppers preferred immediate consumption.
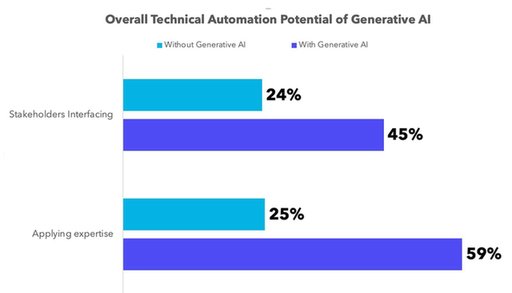
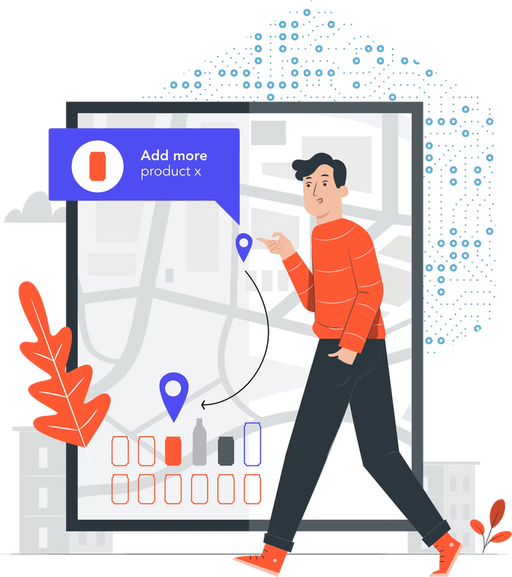
AI and data were employed to test these assumptions, leading to an experiment that assessed the feasibility and potential impact of shifting larger pack sizes of domestic premium brands from the cold to the warm planogram. The experiment revealed that by moving around 40 percent of the Points of Distribution (POD) of a brand, specifically the larger pack sizes, would generate a 13.7% increase in overall category revenue and an amazing 9.4% increase in revenue for our client's brand. This shift opened up a promising revenue opportunity and maintained a feasible plan for store layouts.
The experiment validated the initial hunch about the domestic premium shopper, leading to strategic decisions that respected the buyer's original decisions and maintained the integrity of the store layouts. This case study demonstrates the power of AI in turning intuitive hunches into actionable strategies, opening up new avenues for assortment plans, and overcoming space constraints. It's a testament to the potential of store-level insights in revolutionizing retail, challenging old assumptions, and presenting new perspectives for increasing category growth.
Case Studies: Real-World Applications of AI in Portfolio, Assortment, and Space Planning
Case Study 1: Optimizing Assortment Space Contribution Index with AI
The Space Contribution Index (SCI) is a critical assortment planning and optimization metric. It measures the proportion of linear shelf space a product occupies relative to its sales performance. However, calculating the SCI can be complex, especially considering the vast amount of store-level data that needs to be analyzed.
AI and advanced data analytics techniques have been used to streamline the calculation of the SCI. By leveraging AI algorithms with store-level data, retailers can get a much more granular and accurate picture of their product assortment optimization. This leads to better decision-making and more effective shelf space allocation, regardless of the number of store clusters analyzed.
For example, a retailer looking to optimize its cereal category used AI-driven clustering analytics to identify patterns and trends in store-level data. The retailer had over 18,000 stores in the continental United States with just four clusters. After analyzing all four clusters, significant suboptimal indexing was observed, ranging from as low as 45% to over-indexing at 145%. By understanding this with AI's speed, the retailer could develop targeted recommendations for reallocating space based on specific parameters such as minimum sales thresholds or store door count.
Case Study 2: Tailoring Assortments to Individual Stores with AI
Understanding each store's unique needs and preferences is crucial for maximizing sales. AI offers the ability to prepare store-specific planograms based on store-level insights.
A recent analysis of 400 stores discovered that by using AI to create store-specific assortments, 8% of the stores (33 stores) could see an increase of over 15% in total incremental sales. However, as the retailer expanded store-specific assortments to more stores, the contribution to incremental sales began to decrease.
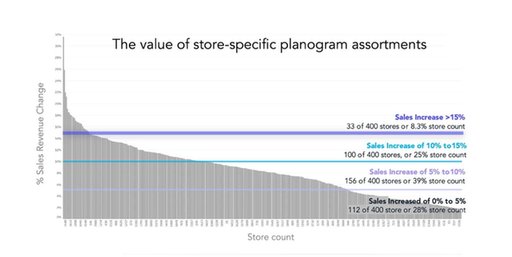
This analysis revealed a critical insight: while store-specific planograms can drive significant growth, it's crucial to select which stores to focus on strategically. In this case, the 33 stores with a potential sales increase of over 15% would be the best candidates for store-specific planograms. These stores likely have a unique shopper profile that can be effectively targeted with a tailored assortment.
Case Study 3: Informing Price Pack Architecture with AI
The pack size and brand preferences vary among different retailers like Walmart, Target, and Dollar General. After analyzing several brands of a multinational CPG customer, we discovered that certain brands and pack sizes are crucial for different retailers. For instance, Target customers prefer large packs, while Dollar General shoppers prefer miniature packs, and Walmart dominates most with one pack size. With this insight, our AI- powered model can create space-aware strategies to design optimal category plans for various retailers, increasing revenue and volume while considering our client's brand portfolio by retailer. This discovery has helped us gain insights into specific distributors, allowing us to determine the ideal mix for each retailer with the help of AI.
Case Study 4: Optimizing Days of Supply with AI
AI can optimize the standard deviation around Days of Supply (DOS) for categories. The AI model was used to understand the POD (Points of Distribution) of a specific category at the store level to see if the wide spread of DOS between 5 to 1 day; could be optimized or at least reduce the spread. After applying a store-level approach, the AI was able not only to reduce the spread and ensure no stores had a DOS of 2.5 days or less. The study underscores the role of AI in balancing inventory, driving sales growth, and reducing waste by adjusting product facings on the shelf and retaining essential items in specific stores.
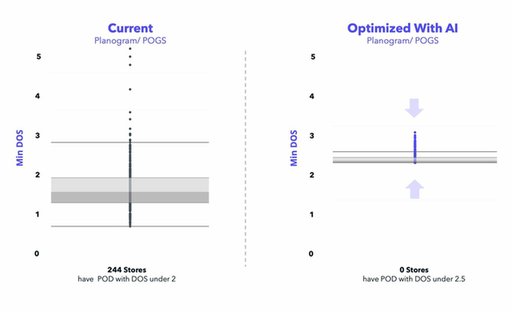
The Future of Retail: AI as a Co-Pilot for Strategic Decision-Making
As the industry navigates through this complex landscape, AI and advanced analytics are emerging as powerful tools to help retailers adapt and thrive.
AI is reinventing the role of retail merchants by automating mundane, data-driven tasks and providing predictive insights. This shift lets retailers focus more on strategic decision-making and less on routine tasks. AI is also helping to break down functional silos within retail organizations, enabling a more holistic view of enterprise-wide data and insights.
One of the key benefits of AI in retail is the ability to create a granular customer view. This enables retailers to understand customer profitability, loyalty, and buying behavior for each interaction. With this insight, retailers can optimize assortments, channels, and space to delight customers and drive growth.
AI also empowers retailers to turn data into responsible actions quickly. Predictive, prescriptive insights and detailed scenario simulations allow retailers to test and learn more quickly, refining their strategies based on data-driven insights.
Moreover, AI is enabling new business models in retail. Retailers can monetize their data and share key insights with valued suppliers, establishing marketplace platforms with optimized customer targeting. This not only creates new revenue streams but also strengthens relationships with suppliers.
Embracing the AI Revolution in Retail
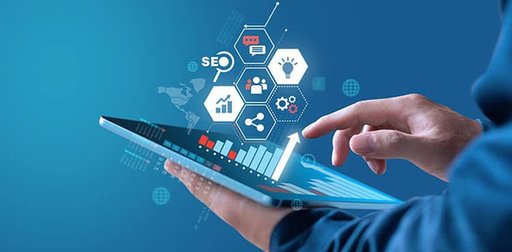
As we navigate the rapidly evolving retail landscape, it is clear that integrating AI and advanced analytics into retail strategies is not just an option but a necessity. The ability to leverage store-level data and transform it into actionable insights is revolutionizing how retailers and CPG suppliers operate, enabling them to make more informed, strategic decisions that drive growth and profitability.
The power of AI lies in its capacity to analyze vast amounts of data at an unprecedented speed and accuracy, providing granular insights that were previously unattainable. This allows for a deeper understanding of customer behavior, enabling retailers to tailor their product assortments, innovate effectively, and optimize their category strategies. The result is a more personalized shopping experience that meets customers' needs while maximizing sales and profit.
Moreover, AI empowers retailers and CPG suppliers to challenge old assumptions and test shopper insights, presenting new perspectives for increasing category growth. It turns intuitive hunches into data-driven strategies, ensuring that decisions are based on solid evidence rather than guesswork.
The case studies presented in this report demonstrate the transformative potential of AI in retail. They show how AI can identify patterns and trends in store-level data, optimize retail space, validate shopper assumptions, and even determine the value of store-specific planograms. These are powerful demonstrations of how AI can turn insights into actionable strategies for retail success.
As we look to the future, it is evident that AI will continue to play a pivotal role in retail. As your co-pilot, AI can help navigate the complexities of the retail landscape, providing the tools needed to stay competitive in an increasingly data-driven world. Embracing the AI revolution in retail is not just about keeping up with the times; it's about paving the way for a more efficient, profitable, and customer-centric future.
The AI revolution in retail is here, and it's time to embrace it. By harnessing the power of store-level insights, retailers and CPG suppliers can unlock new opportunities, drive innovation, and pave the way for success in the modern retail landscape. The future of retail is data-driven, and with AI as your co-pilot, the possibilities are endless.