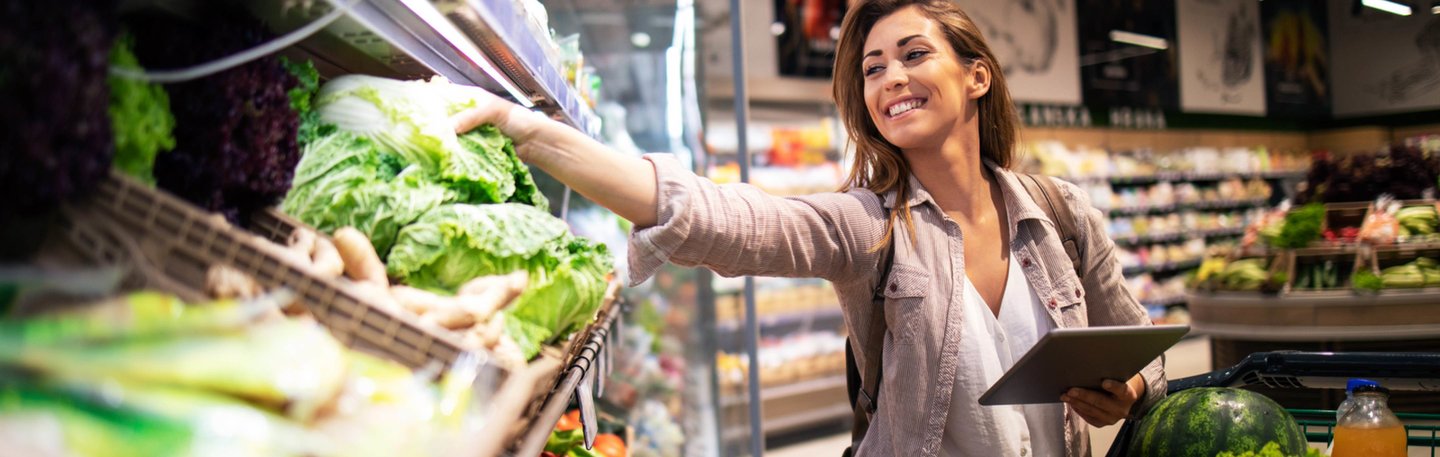
What is wrong with today’s demand forecasting methods for merchandising & assortment planning?
By Matt Schilb - VP, Product, HIVERY
Today’s demand forecasting methods are not equipped to deal with the complexity of today’s retail environment. If you think about it, retailers and CPG manufacturers work together to:
- Examine thousands of SKU combinations across different categories
- Analyze historical sales data, and
- Look at the complex space and localization needs of each SKU for each store.
They must do this while determining the impact of demand transfer and product innovation, staying mindful of all the real-world merchandise rules and constraints. All while maximizing their revenue, volume, or profit! That is a lot to consider.
Demand forecasting is about how much product will be sold in the future and is used by retailers and manufacturers for just about everything...from merchandise planning to replenishment and allocation decisions. Demand forecast offers important insight and directional strategies; however, it can be a relatively blunt instrument. It is not equipped to handle the precision needed to make operationally efficient and locally relevant decisions.
The traditional approach to forecasting has been based on statistical methods that experts in the field primarily develop. An AI/ML approach flips this upside down.
By Matt Schilb - VP, Product, HIVERY
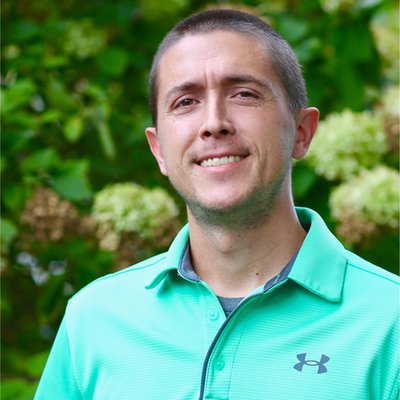
We need to go beyond standard demand forecasting methods and leverage the power of AI/ML to generate predictions that consider the impact of an ever-growing number of SKUs, the unique characteristics of every store, and associated goals and constraints. Advanced methodologies have the potential to not just “predict” assortment demand and transfer; they can simulate the impact of any merchandise cluster strategy. You want to go beyond legacy demand forecasting and leverage systems/methodologies that seamlessly toggle between strategic analysis and operational execution.
Forecasting Is Expensive
Forecasting is typically the most data-intensive, time-consuming, and expensive part of planning. You often need to rely on data engineering and data science teams for critical data input and other stakeholders (brand marketing, sales, and category management teams). Once you have the data, you generally use something like Tableau, Power BI, or Excel to generate forecasts. While you can do some basic “what if” plays, these are often not grounded by the reality of execution complexities, and you often make assumptions.
Collaboration can be Challenging and Slow
Since you rely on many stakeholders and the analysis can take days, if not weeks, you cannot rapidly pose new questions and ask different strategic questions. If you do, you need to re-run the numbers and re-do the analysis. Often you might need to input new datasets. This makes collaboration extremely slow and difficult, hindering your assortment and space planning later.
Advanced methodologies have the potential to not just “predict” assortment demand and transfer; they can simulate the impact of any merchandise cluster strategy.
Strong assortment decisions need granularity at store level, with the flexibility to roll up into different store cluster strategies. The problem with current demand planning is that category, and assortment planning teams often make decisions on a top-down approach. Predictions are based on “averages of averages” and focus on the top performing items at a national level. This means you’re not getting the right SKUs to the right stores or store clusters.
Many Legacy Methods Don’t Consider Store-Level Needs
Time Series for Demand Planning Doesn’t Adapt
Common demand forecasting methods like time series analysis involve looking at historical data to predict future demand. This approach relies on the assumption that future behavior will be constant. In reality, many variables need to be considered. In fact, you are constantly juggling a range of variables and wondering: what SKUs are there that I do not want? What SKUs are not there but need to be? What space should I allocate to those products and in which stores?
Unplanned market shocks like COVID-19, ongoing changes in shopper behavior, supply chain issues, and evolving trends mean you need answers that can be dynamic and fast! Your assortment planning should respond to market changes quickly. Today's demand forecasting methods cannot "learn over time," and once the data is extracted and analyzed, the analysis is outdated.
Further methods like time series do not (and cannot) consider real-world assortment/merchandise rules, constraints, and category objectives that the business is trying to manage in their modeling. Nor can they detect new data relationships or patterns within large datasets. They have also inherited human dispositions from sites such as anchor bias.
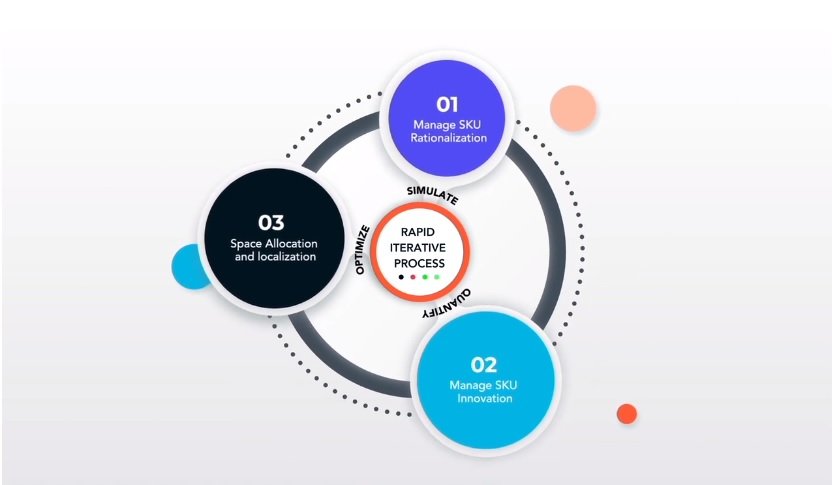
Is there a better approach?
Simulation powered by AI/ML closes these gaps and allows for real-time analysis \across any number of desired scenarios.
AI/ML Approach Opens Up New Doors
The traditional approach to forecasting has been based on statistical methods that experts in the field primarily develop. An AI/ML approach flips this upside down and says, "Hey AI, you tell me what you see from the dataset I give you." Once the algorithmic model is designed, and training is completed using a sample of the data, the model is dynamic.
In fact, recent advances in AI/ML have enabled us to create models that can learn from historical data on their own and adjust their own error predictions based not only on past datasets but, more importantly, the relationship or patterns found within those datasets.
Since an AI/ML approach ultimately creates a “trained model” of the world, you can start posing questions; running simulations (or "scenarios") on those questions while incorporating any merchandise rules, constraints, and category objectives - all at the store level. The dynamic nature of the model means you can interact with it at any time, pose a new assortment of strategy questions, and get rapid answers. You do not need to rely on your BI or data science teams. Since the time gap between question and answer is dramatically reduced, you can collaborate with internal and external stakeholders in ways not normally possible.
Further, since the model can seamlessly use store-level datasets, it can provide store-specific SKU selection granularity in its recommendations or at any cluster count you need. This means you CAN get the answers to questions like:
- What is the cost of an incremental facing?
- What is the impact of going neutral on Points of distribution (POD)?
- What are the best stores to sell our innovation items but not impact our flagship brand?
- How to determine the fiscal impact of changing merchandising styles at each SKU item at the store level - including allowing for different SKU orientations, fixtures,s and stacking heights?
But now, you can go even further and discover new store cluster strategies:
- What is the value of going store-specific?
- What is the opportunity to re-optimize the existing clusters strategy?
- How can I optimize my days of supply strategy to reduce out-of-stock and meet the local demands of each store?
Demand Forecasting Is Limited. Simulations Are Smarter
In close, simulations go a lot further than legacy demand forecasting methods. And they consider real work rules and constraints while optimizing for your category/assortment goals. This means that any optimized assortments and planograms are executable at store level (or clusters of stores).
Collaborate and equate every decision with your buyer speed and transparency while delivering locally relevant, effectively merchandised, and operationally efficient assortments.
Luc Filiatreault is the CEO of mdf commerce, a company providing eprocurement and unified commerce B2B SaaS solutions to optimize and accelerate commercial interactions between buyers and sellers, servicing over 600 clients in eCommerce alone. Luc has founded and/or led seven businesses in technology, IT and aerospace, completed two IPOs, and raised multiple levels of funding totaling over 200 M$ from Canadian and US venture capitals, as well as institutional investors.